Search and Recommendations
Platforms that generate search results and recommendations share the common goal of optimizing relevance. This is measured by engagement actions such as clicking on a search result or recommendation.
Typical systems have models that estimate the click probability or relevance of search results or product recommendations.​ When returning a group of results, the algorithm typically returns the links with the top scores. This is sub-optimal. A better way is to optimize the group of links together. ​
A common mistake is to return links with nearly identical content are often returned. ​ To a certain extent content deduplication helps mitigate this problem. However, this approach still fails to consider content that is similar but still different.
How should you manage the trade-off between similarity and difference? Furthermore, how should you treat content with uncertain or unknown relevance? ​Quante Carlo solves this problem with Bayesian Optimization.
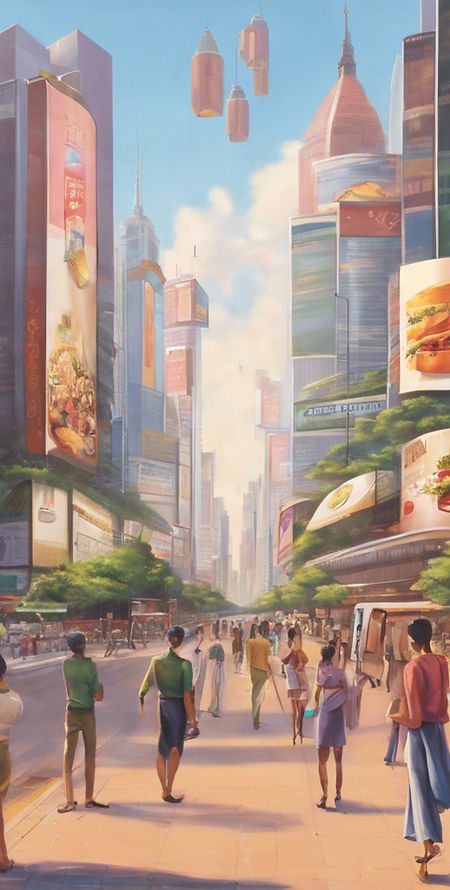